How Protein Folding Softwares Revolutionized Medicine
- Bruins for Business Health at UCLA
- Feb 18
- 7 min read
By Khalif Kamil
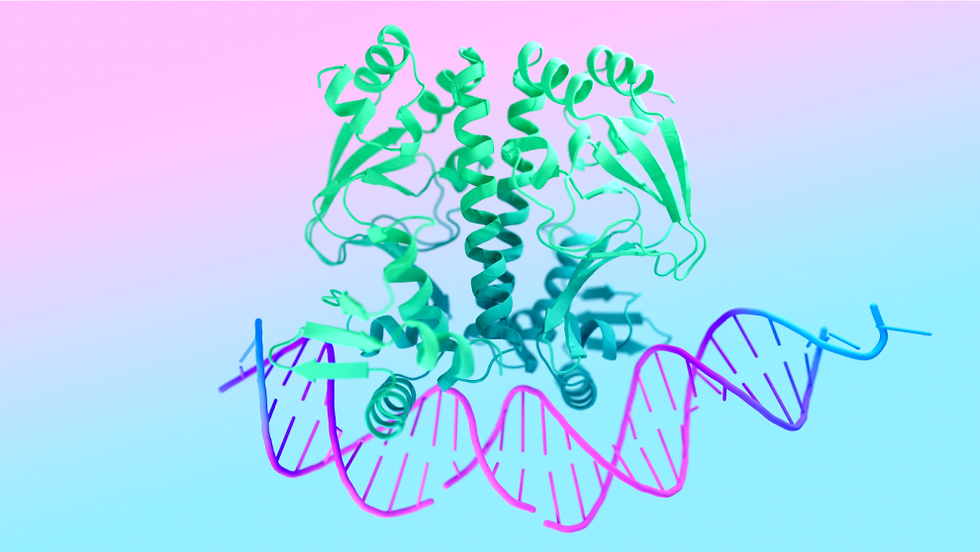
Background
Proteins are complex molecules that perform a wide array of functions in living organisms. These workhorses of biology are crucial to life, from providing structural support, to facilitating chemical reactions as enzymes, to transporting molecules, and beyond. A protein’s function and capability is determined by their 3-D structure that occurs after the folding of amino acids, the building block proteins. However, misfolded proteins can prevent proper functioning in the body and even lead to disease. Thus, the importance of understanding protein structure and protein folding is exemplified. In other words, understanding how a protein folds can provide a link between its genetic code, structure, and function. Traditional methods to determine protein structures are expensive, time-consuming, and sometimes impossible for certain proteins. In addition, accuracy can vary across different methods and proteins. This problem is known as the “protein-folding problem,” and has been studied by biologists for decades.
The protein-folding problem is a fundamental challenge in biology, centered on predicting a protein’s three-dimensional structure based on its amino acid sequence. Proteins perform critical functions, which depend on their unique shape. Protein structure is determined by the folding process—a complex and dynamic arrangement of the amino acid chain into a stable 3D structure. While the sequences of many proteins are known, determining their structures has traditionally required labor-intensive and expensive experimental techniques, such as X-ray crystallography. Misfolded proteins can lead to diseases like Alzheimer’s, Parkinson’s, and certain cancers, making this problem not just a theoretical question but one with profound implications for health and medicine. Solving the protein-folding problem may revolutionize drug discovery, enzyme design, and our understanding of cellular processes. As a result, many computational biologists have sought to develop software that can tackle the protein-folding problem.
Protein Folding Software
Scientists began using computer programs to predict protein shapes in the 1980s, employing novel technologies to tackle this pressing challenge. However, early computational models relied on simplified physics-based methods, which were limited by computational power and available experimental data. The technology available and scientific knowledge on proteins were simply insufficient to actually create accurate software. With the rise of bioinformatics, scientists were able to gather more data and experiment with different protein structures. For example, in the 1990s and early 2000s, databases like the Protein Data Bank (PDB) provided valuable experimental data for modeling. Later, early algorithms like Rosetta, a software developed by David Baker, improved the accuracy of predictions but were still far from accurate. These programs began using more sophisticated techniques and were tested on larger data banks, contributing to improvements in the software over time.
CASP Competition
How were these softwares being rated and evaluated? Who was in charge of guiding these programs and their accuracy? The baseline standard for evaluating accuracy of protein-folding softwares is the CASP. The Critical Assessment of protein Structure Prediction (CASP) competition, launched in 1994, judges computational methods for predicting protein structures. Groups that participate, funded by either universities, research institutions, or private companies, are given the challenge of predicting the 3D structures of proteins from just their amino acid sequences. This challenge introduced a competitive aspect for scientists, pushing research groups to compete with one another and develop more advancements.
As mentioned earlier, most computational predictions were initially very inaccurate, as they were based on relatively simple models and low computational power, compared to today. However, researchers made great strides over the next few decades, in large part due to techniques that utilized novel biochemistry principles, such as molecular threading. In addition, computer softwares and networks progressed, and the software had a greater array of proteins to test on. Coupled with the rise of bioinformatics as a field, many scientists developed artificial neural networks that could analyze data in the Protein Data Bank more efficiently. Despite these improvements, methods still lagged behind the desired experimental results.
AlphaFold and CASP
With rapid strides in machine learning in the 2010’s, artificial neural networks seemed poised to finally solve the “protein-folding question”. The AlphaFold software, developed by DeepMind, used machine learning methods to improve accuracy, achieving near-experimental accuracy for many targets for the first time. It was first introduced in CASP 13, in 2018, and was the first entry to heavily utilize artificial intelligence in its computational software, garnering a mean accuracy score of 68.5%. This revolutionized the field by leveraging deep learning and neural networks, capable of analyzing vast datasets of known protein structures and sequences. Relentless pursuit of innovation led to more breakthroughs in subsequent CASP competition softwares.
AlphaFold 2 was developed by DeepMind in 2020 for the CASP 14. AlphaFold 2 built upon and improved the existing neural network developed earlier. Through artificial intelligence, they once again achieved near-experimental accuracy (92%) in predicting protein structures, outperforming other methods by a wide margin. A key concept employed in AlphaFold was deep learning. Deep Learning is a specific type of machine learning that can learn from its mistakes, identify patterns, and use artificial neural networks to continually improve it. This time, it solved previously unsolved structures with unprecedented precision.
AlphaFold 2 and other AI-driven approaches now provide highly accurate predictions for a vast majority of protein structures, surpassing human expertise in many cases. These advancements have drastically reduced the time and cost required to determine protein structures, opening up new possibilities for drug design, disease understanding, and biotechnology. AlphaFold’s predictions are now freely available, covering nearly the entire human proteome and many other species. In addition, the creators made it Open Source, so anyone is able to access and use the software, results, and proteins, further driving biotech innovation.
CASP has played a crucial role in driving the field forward, from initial, rudimentary predictions to the groundbreaking achievements of modern AI-based systems like AlphaFold, which now dominate the competition. Now, most entries in CASP actually use AlphaFold software or a variant of it. AlphaFold is considered a milestone in computational biology, enabling scientists to solve previously intractable problems and dramatically reducing the time and cost required for protein structure determination.
Beyond AlphaFold/Other Softwares:
Competitors like RoseTTAFold (developed by David Baker’s lab) and OpenFold continue to refine the field. David Baker had previously made “Rosetta” which preceded AlphaFold and was the most accurate software in the 2000’s.
AlphaFold was made open source in 2021, and in CASP15 in 2022. While DeepMind did not enter, virtually all of the high-ranking teams used AlphaFold or modifications of AlphaFold. In addition, AlphaFold’s database has also been made public, and contains over 200 million protein structures, allowing researchers to test and train new softwares on a vast array of proteins.
The next step is exemplified through the efforts underway to predict not just static structures, but dynamic processes, interactions, and protein-ligand complexes. This holds a key difference from the previous “protein-folding problem”. Instead of analyzing the still structure of a protein, researchers want to predict how structures of molecules change over time, during certain processes and interactions.
Use Case
Researchers at the University of Toronto and Insilico Medicine, a biotechnology company, used AlphaFold to create a cancer drug. Remarkably, this drug was developed in under 30 days, from discovery of a protein “weak spot” to drug creation and testing.
The researchers used AlphaFold and its vast database to analyze hundreds of proteins and their structure. Used in tandem with an in-house AI, they detected a “weak spot” in a specific protein associated with hepatocellular carcinoma, a type of liver cancer. A protein “weak spot” is simply an area that is more susceptible to disruption or unfolding, and thus could be targeted with a drug. AlphaFold was used specifically to more accurately predict the shape of the protein and its weak spot. Michael Levitt, a Nobel Prize-winning chemist and member of Insilico’s board, specifically highlighted AlphaFold’s ability to scan through several proteins broadly for weak spots, connecting information that humans may otherwise miss. Using AlphaFold’s generated protein structure, researchers created a drug that inhibits this protein. This drug was able to slow cancer growth in cultured cells.
Awards and Outlook
The importance of unlocking protein folding cannot be understated. In fact, three scientists that worked on AlphaFold were just awarded the Nobel Prize in Chemistry for their contributions to computational protein design and protein structure prediction. In just a few years, many medical advancements have been made due to AlphaFold and similar softwares. Their breakthroughs solved one of the longest-standing problems in biology, leveraging chemistry and AI to do so.
The future of protein-folding AI in medicine holds transformative potential. As these technologies advance, they could revolutionize drug discovery by designing targeted treatments tailored to individual protein structures, improving effectiveness and reducing side effects. AI could also help identify new disease biomarkers for earlier diagnosis, particularly for conditions linked to protein misfolding, like Alzheimer’s and Parkinson’s. Additionally, AI may enable the creation of novel therapeutic proteins and enzymes for rare genetic disorders and environmental challenges. With faster, cheaper drug development, AI-driven protein folding could dramatically accelerate the search for cures, making advanced, personalized treatments more accessible.
Works Cited
Abramson, J., Adler, J., & Dunger, J. (2024). Accurate structure prediction of biomolecular
interactions with alphafold 3. Nature, 630(8016), 493–500. https://doi.org/10.1038/s41586-024-07487-w
Dill, K. A., & MacCallum, J. L. (2012). The protein-folding problem, 50 years on. science,
338(6110), 1042-1046.
Jumper, J., & Evans, R. (2021). Highly accurate protein structure prediction with alphafold.
Nature, 596(7873), 583–589. https://doi.org/10.1038/s41586-021-03819-2
Ren, F., Ding, X., et al. (2023). Alphafold accelerates artificial intelligence powered drug
discovery: Efficient discovery of a novel cdk20 small molecule inhibitor. Chemical Science, 14(6), 1443–1452. https://doi.org/10.1039/d2sc05709c
Schreiner, Maximilian (2022-12-14). "CASP15: AlphaFold's success spurs new challenges in
protein-structure prediction". The Decoder. Retrieved 2023-02-13
Straiton, J. (2023). The Path to Solving the Protein Folding Problem. BioTechniques, 74(5),
Yang, Z., Zeng, X., Zhao, Y. et al. (2023). Alphafold2 and its applications in the fields of biology and medicine. Signal Transduction and Targeted Therapy, 8(1). https://doi.org/10.1038/s41392-023-01381-z
Comments